Project Description: Stochastic modelling and graphical models for the analysis and prediction of phenotype interactions
Posted by: Melanie 11 years, 4 months ago
This entry describes one of the projects sponsored by the MLPM-ITN scholarship.
Problem Overview
In the past decades, the emergence of new technologies has lead us to a true data explosion in all areas, including the field of medicine. Although we are able to collect a huge amount of data from patients, we are still far from processing all this valuable information to provide a truly personalized medical treatment. In order to solve this problem, our aim is to design statistical/machine learning models that help physicians better understand diseases and their complex interactions.
Our current focus lies on the analysis of psychiatric disorders and their underlying interactions. Indeed, psychiatric disorders are known to have a high comorbidity, i.e., different disorders tend to co-occur at a higher frequency than chance.
The problems that we are considering are the following:
- be able to detect the different psychiatric patterns.
- model the existing correlation across observations given a certain disorder.
- infer the respective relationships between different disorders.
All these problems will be addressed following a Bayesian Nonparametric Approach (see description below).
Current Research
In order to find the different psychiatric disorders (problem 1.), we consider both a latent mixture model, using a Dirichlet Process (DP) prior, as well as a latent feature model, using an Indian Buffet Process (IBP) prior for discrete observations.
Problem 2. consists in incorporating correlation into the observation model. This information would help psychiatrists to measure the amount of information provided by each new question, given the all the previous answers. In order to do so, correlations are modeled as communication noise channels that are also inferred in our BNP model.
Finally, problem 3. considers correlation at the level of latent variables, and will be considered in the future.
Bayesian Nonparametric Models
Bayesian Nonparametrics (BNP) has been an active field of research in the last decade. Such models have been used to address a wide variety of problems, such as classification, regression, clustering or collaborative filtering.
The Bayesian approach allows us to include all the available information a priori, which makes our model more robust, interpretable, and consistent with reality. Additionally, the Nonparametric property makes such methodology very flexible, for the solution complexity depends on the available amount of data.
If we have little data, the number of parameters will be small and simple solutions will be found, avoiding overfitting. But if many input samples are available, the nature of the problem will be more complex, and the number of parameters will automatically grow as necessary, avoiding model selection. This feature allow us to explain novel aspects of the data that were so far unknown.
Information about the Database used
For this purpose, we have a large amount of information in the National Epidemiologic Survey on Alcohol and Related Conditions (NESARC) database. This database collects data on the background of participants, alcohol and drug usage, behavioral and personality traits. We are currently using Wave 1 of this database and we are trying to gain access to Wave 3 in which there is genetic information for the subjects.
Recent Posts
- MLPM2012 has ended – thank you!
- Final ITN meeting and Krupp Symposium in Munich
- MLPM ITN fellow Melanie brings science to classrooms and inspires with simple but exciting experiments
- ESHG Symposium – a great success!
- Team working event: The 2nd ITN March retreat
Archive
2017
- January (1)
2016
2015
2014
2013
Categories
- Awards (2)
Authors
- Melanie (1)
- Felipe (1)
- MLPM-Admin (22)
- MLPM (2)
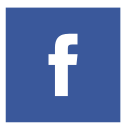
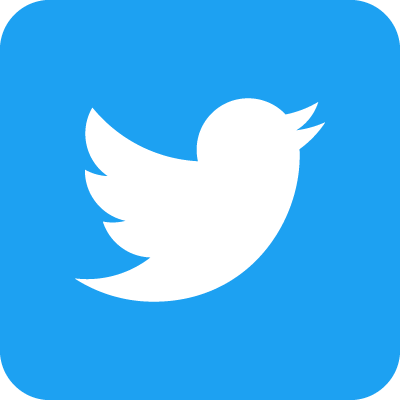