Talks and speakers
GUEST SPEAKERS:
Robert Castelo
robert.castelo@upf.edu
Systems genetics with graphical Markov models
High-throughput genomic profiling instruments provide a snapshot of the simultaneous activity of molecules within cells. The resulting readouts, obtained in parallel for thousands of different functional elements in the genome, have enabled the analysis of cellular pathways at the systems level. A simple, fundamental and primary type of such an analysis consists of assessing changes across experimental conditions independently in each molecular profile. Yet, the fact that these data constitute a multivariate sample conveys the opportunity for us to gather additional insight by examining direct and indirect effects between genome elements such as genes and mutations. Graphical Markov models (GMMs), developed at the crossroads of graph theory, machine learning and statistics, are a sensible approach to pursue this goal. In this talk I will introduce GMMs and our recent work on how to use them to study the genetics of gene expression using the software qpgraph, developed in our group. I encourage the audience to bring along their laptops with the latest version of R (http://www.r-project.org) and the Bioconductor package qpgraph (http://bioconductor.org/packages/qpgraph) installed, to try to work out together some of the examples given during the talk.
> The speaker kindly provided the SLIDES of his talk.
> You can watch the talk here.
******************************************************************************************
Krista Fischer
Unlocking the potential of large prospective biobank cohorts for -omics data analysis: aspects of study design, prediction and causality
Recent decade has seen a tremendous increase in availability of data from large population-based biobank cohorts. Such datasets include various types of -omics data (genomics, transcriptomics, metabolomics etc) as well as extensive data on participants' health, lifestyle and demographics at recruitment and often also detailed follow-up data from electronic health registries and other databases. This talk will discuss aspects of study design and statistical analysis based on such datasets.
First of all, the options of analysis of follow-up data will be discussed, in order to evaluate potential -omics based predictive biomarkers. One important issue to consider is the choice of timescale. Unlike traditional survival analysis projects, the recruitment time does not mark any important event (such as diagnosis of a serious disease) in the participants life course and therefore the actual follow-up time may not be the optimal timescale to use. However, this depends also on types of biomarkers to be considered - whether they depend on current health status of the participant (as metabolomics data, for instance) or are determined at birth (DNA-based markers). We illustrate the concepts based on both simulated data and the Estonian Biobank cohort to understand, what is the optimal analysis strategy in each of the situation considered. Another issue is study design - especially in cases where only a subset of a large cohort can be selected for genotyping or another kind of sample processing to obtain the relevant -omics data. Here, the potential of nested case-control study design will be discussed.
The second topic to be discussed is the use of genetic data in personalized risk prediction. Large biobank cohorts provide data to compare and validate such predictors. In case of common complex diseases, the polygenic nature of the disease has to be taken into account and therefore multimarker scores have considerably better predictive ability than any of the single SNPs. Here it is important to reach on optimal decision on the choice of genetic markers to the score as well as the weights used to combine them. The concept will be illustrated using the example of Type 2 Diabetes risk prediction in the Estonian Biobank data.
Finally, some aspects of causal modeling will be discussed. Availability of large cohorts has encouraged many researchers to use Mendelian Randomization methodology to estimate causal effects of different lifestyle and clinical parameters on the outcomes. However, causal inference techniques always rely on some untestable assumptions and these are often forgotten. We discuss, whether it is possible to distinguish between alternative causal scenarios (such as mediation and pleiotropy) in case of on genetic and two non-genetic variables.
> The speaker kindly provided the SLIDES of her talk.
> You can watch the talk here.
******************************************************************************************
Lude Franke
University of Groningen, The Netherlands
lude@ludesign.nl
Identifying drug-targetable key drivers of disease
In the last few years genome-wide association studies have revealed over 10,000 genetic risk factors for disease. For many disorders it is now clear that there are dozens of variants involved, precluding development of drugs that target each of the causal genes in side these loci. However, since per disease these variants typically affect a limited number of pathways, devising strategies to uncover the ‘key driver’ genes and pathways for these diseases might provide leads for pharmaceutical intervention. By combining co-regulation networks (Fehrmann et al, NG 2015), trans-eQTLs (Westra et al, NG 2015), trans-meQTLs (Bonder et al, BioRXiv 2015) and novel analytical methods (Depict et al, Nature Communications 2015, Zhernakova et al, BioRXiv 2015) we believe these key driver genes might be uncovered. I will discuss these approaches and will describe how we employ machine learning to answer the questions that we work on.
> The speaker kindly provided the SLIDES of his talk.
> You can watch the talk here.
******************************************************************************************
Luis Serrano
Centre for Genomic Regulation (CRG), Barcelona, Spain
luis.serrano@crg.eu
Integrative and quantitative analysis of disease mutations in the RAS-RAF-MEK-ERK pathway and implications for personalized medicine
The Ras/MAPK syndromes ('RASopathies') are a class of developmental disorders caused by germline mutations in 15 genes encoding proteins of the Ras/mitogen-activated protein kinase (MAPK) pathway. It is intriguing that mutations in the same 15 genes are also frequently identified in different types of human cancers. In this talk, I will shed light on 956 RASopathy and cancer missense mutations by combining protein network data with mutational analyses based on 3D structures [1]. Using the protein design algorithm FoldX and mathematical network modelling, we show that quantitative rather than qualitative network differences determine the phenotypic outcome of RASopathy compared to cancer mutations. Furthermore, our quantitative predictions can explain why some cancer mutations (‘drivers’) occur at significantly higher rates than - presumably - functionally alternative mutations. For example, V600E in the BRAF hydrophobic activation segment (AS) pocket accounts for >95% of all kinase mutations. We used experimental and in silico structure-energy statistical analyses, to elucidate why the V600E mutation, but no other mutation at this, or any other positions in BRAF's hydrophobic pocket, is predominant. We find that oncogene mutations frequencies depend on the equilibrium between the destabilization of the hydrophobic pocket, the overall folding energy, the activation of the kinase and the number of bases required to change the corresponding amino acid [2]. Using a random forest classifier, we quantitatively dissected the parameters contributing to BRAF AS cancer frequencies. These findings can be applied to genome-wide association studies and prediction models.
[1] Kiel C, Serrano L. Structure-energy-based predictions and network modelling of RASopathy and cancer missense mutations. Mol Syst Biol. 2014 May 6;10:727
[2] Kiel C, Benisty H, Lloréns-Rico V, Serrano L. The yin-yang of kinase activation and unfolding explains the peculiarity of Val600 in the activation segment of BRAF. Elife. 2016 Jan 8;5. pii: e12814.
> The speaker kindly provided the SLIDES of his talk.
> You can watch the talk here.
******************************************************************************************
Terry Speed
Bioinformatics Division, Walter and Eliza Hall Institute of Medical Research, Australia
terry@wehi.edu.au
Removing Unwanted Variation in Machine Learning for Personalized Medicine
Machine Learning for Personalized Medicine will inevitably build on large omics datasets. These are often collected over months or years, and sometimes involve multiple labs. Unwanted variation (UV) can arise from technical elements such as batches, different platforms or laboratories, or from biological signals such as heterogeneity in age, ethnicity or cellular composition, which are unrelated to the factor of interest in the study. Similar issues arise when the goal is to combine several smaller studies. A very important task is to remove these UV factors without losing the factors of interest. Some years ago we proposed a general framework (called RUV) for removing UV in microarray data using negative control genes. It showed very good behavior for differential expression analysis (i.e., with a known factor of interest) when applied to several datasets. Our objective in this talk is to describe our recent results doing similar things in a machine learning context, specifically when carrying out classification.
> The speaker kindly provided the SLIDES of his talk.
> You can watch the talk here.
******************************************************************************************
Alfonso Valencia
Spanish National Cancer Research Centre (CNIO), Madrid
valencia@cnio.es, www.cnio.es, @alfons_valencia
A Network Biology Approach to Epigenetic Regulation
The description of molecular systems as networks opens the possibility of using all the methodology developed for network analysis in other fields from sociology to neurology. In this case, we have analysed the properties of Mouse Embryonic Stem Cells (mESC) at different levels of the organization of epigenetic modifications. mESC is currently the best characterised epigenetics system, including data on many of the basic components: Chromatin Related Proteins (CRPs), Histone modifications, DNA methylation modifications, the genome mapping preferences of a large collection of proteins and modifications (ChIP-Seq data) and organization of the chromatin in the nucleus, determined with Chromatin Capture Experiments.
We have processed these heterogeneous “mESC Epigenetic Properties” to build a comprehensive network of CRPs, histone marks and DNA modifications linked by their propensity to co-localize in the genome. In this network co-localization preferences are specific of “mESC Chromatin States”, such as Promoters and Enhancers. The analysis of the properties of the “co-localization” network points to one of the DNA modifications 5hmC as the key component in the organization of this network. The importance of 5hmC in the network is reinforced by the evolutionary analysis of the protein components of the network, in which 5hmC acts as a mediator in the co-evolution of the protein components of the mESC network.
We have further explored the functional significance of the “mESC Epigenetic Properties” and “mESC Chromatin States” by analysing them in the context of the structure of the nucleus that ultimately controls genes expression. The results revealed interesting properties of the organization of the mESC epigenetic control system, in line with the emerging models of gene expression control and chromatin organization. At the methodological level, I will introduce the growing importance of Network analysis methodology in the exploration of the functional and evolutionary properties of complex biological systems.
- Epigenomic Co-localization and Co-evolution Reveal a Key Role for 5hmC as a Communication Hub in the Chromatin Network of ESCs. Perner et al., (2016) Cell Rep. http://www.cell.com/cell-reports/pdf/S2211-1247(16)00028-0.pdf
- Chromatin assortativity: integrating epigenomic data and 3D genomic structure. Pancaldi et al., (2016) http://arxiv.org/abs/1512.00268
- This work was developed in collaboration with the Vingron's (MPIMG, Berlin) and Fraser’s (Babraham Institute) labs, and it was financed in part by the BLUEPRINT consortium (http://www.blueprintepigenome.eu)
> The speaker kindly provided the SLIDES of his talk.
> You can watch the talk here.
******************************************************************************************
SPEAKERS OF THE INITIAL TRAINING NETWORK "MACHINE LEARNING FOR PERSONALIZED MEDICINE" (ITN MLPM)
A short overview of the projects of the ITN MLPM will be given by:
- Felipe Llinarez Lopez, ETH Zurich, Switzerland
- Ilaria Bonavita, Max Planck Society, Munich, Germany
- Meiwen Jia, Max Planck Society, Munich, Germany
- Menno Witteveen, ETH Zurich, Switzerland
- Víctor Bellón, ARMINES, Paris, France
- Cristóbal Esteban, Siemens, Munich, Germany
- Max Zwiessele, University of Sheffield, UK
- Daniel Urda Muñoz, Pharmatics Ltd., Edinburgh, UK. You can watch the talk here.
- Ramouna Fouladi, University of Liège, Belgium
- Yunlong Jiao, ARMINES, Paris, France
- Yuanlong Liu, INSERM, Paris, France
- Melanie Fernandez Pradier, University Carlos III, Madrid, Spain
- Cankut Çubuk, Príncipe Felipe Research Center, Valencia, Spain
Find here more informations about the projects of the ITN MLPM.
Recent Posts
- MLPM2012 has ended – thank you!
- Final ITN meeting and Krupp Symposium in Munich
- MLPM ITN fellow Melanie brings science to classrooms and inspires with simple but exciting experiments
- ESHG Symposium – a great success!
- Team working event: The 2nd ITN March retreat
Archive
2017
- January (1)
2016
2015
2014
2013
Categories
- Awards (2)
Authors
- Melanie (1)
- Felipe (1)
- MLPM-Admin (22)
- MLPM (2)
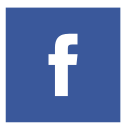
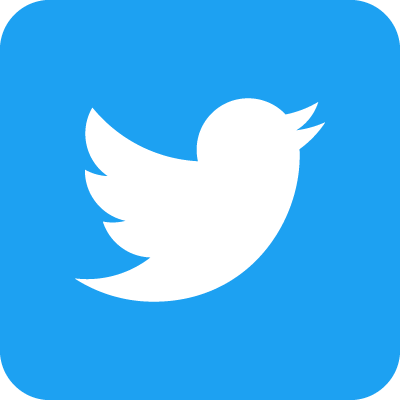