Summer School 2013
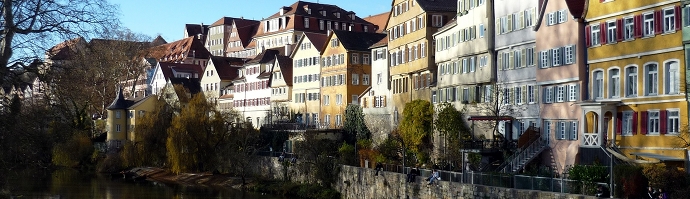
Research Talk by Wolfgang Huber
Part 1: Statistical methods for detecting differential activity from count data in high-throughput sequencing
Many applications of high throughput sequencing require statistical inference based on count data. Mapped reads are often summarised by counting their overlaps with genomic features of interest (genes, exons, binding regions) in samples from different experimental conditions. Applications include differential gene expression, differential exon usage, HiC, ChIP-Seq, CLIP-Seq; similar counting problems are also posed in proteomics. In this talk, I will introduce the use of generalised linear models of the Negative Binomial family for this task, the small-n / large-p problem, empirical Bayes and shrinkage estimation.
Part 2: Complex phenotypes, genetic interactions, automated imaging based phenotyping
Forward genetics uses observational studies of natural phenotypic variation and tries to map the genetic variants associated with phenotypes of interest. A complementary approach is reverse genetics, which introduces controlled, targeted genetic perturbations and tests their phenotypic effects. In many cases, automated image analysis is used to quantify phenotypes. I will discuss the associated machine learning challenges, and also those associated with combining the specific strengths of forward and reverse genetic datasets .