Statistical Significance in Biomarker Discovery
by Karsten Borgwardt
Biomarker discovery is the search for molecular factors that are indicative of disease outbreak, progression or therapy outcome. Due to the polygenic character of many complex phenotypes, it is becoming increasingly important to consider such factors not in isolation, but rather groups and combinations of these factors. Finding such combinations is both a computational and a statistical challenge. The computational challenge arises from the fact that a large space of candidate combinations has to be explored. The statistical challenge is due to each of these candidates representing
a hypothesis that is to be tested, resulting in an enormous multiple testing problem. While there has been enormous effort in making the search more efficient, the multiple testing problem
was deemed intractable for many years. Only recently, new results started to emerge in data mining, which promise to lead to solutions for this multiple testing problem in biomarker discovery. In our talk,
we will present these recent results, including our own work in this direction.
>back to "Talks and Speakers"
Recent Posts
- MLPM2012 has ended – thank you!
- Final ITN meeting and Krupp Symposium in Munich
- MLPM ITN fellow Melanie brings science to classrooms and inspires with simple but exciting experiments
- ESHG Symposium – a great success!
- Team working event: The 2nd ITN March retreat
Archive
2017
- January (1)
2016
2015
2014
2013
Categories
- Awards (2)
Authors
- Melanie (1)
- Felipe (1)
- MLPM-Admin (22)
- MLPM (2)
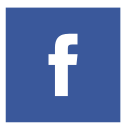
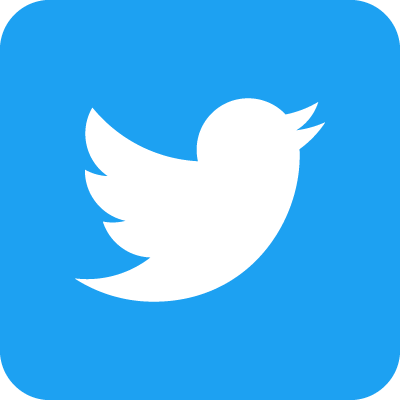