Statistical challenges in the analysis of single-cell transcriptomics data
by Catalina Vallejos
The revolution of transcriptomics - moving from bulk samples to single-cell resolution - can provide novel insights into tissue's function regulation. However, to analyse single-cell RNA sequencing (scRNA-seq) data, methods formulated for bulk experiments cannot be directly applied. In particular, normalisation strategies designed for bulk RNA-seq datasets can lead to unstable results at single-cell level. In addition, the effect of technical variation - reflected in weak correlations among technical replicates - is often ignored by such approaches. In this tutorial, illustrate BASiCS (Bayesian Analysis of Single-Cell Sequencing data) as an analysis tool for scRNA-seq datasets (Vallejos et al, 2015). BASiCS is a hierarchical Bayesian model where (i) normalisation, (ii) quantification of technical variation and (iii) a decomposition of the total variability of gene expression into technical and biological components are performed simultaneously, borrowing information from intrinsic transcripts and technical spike-in genes which are added to the lysis buffer and thence present at the same level in every cell. Adopting such integrated approaches is critical in this context, where an independent analysis of each modelling aspect can veil biological signal. BASiCS also provides an intuitive detection criterion for highly (or lowly) variable genes within the population of cells under study. This is formalised by means of tail posterior probabilities associated to high (or low) biological cell-to-cell variance contributions, quantities that have easy interpretation. We demonstrate BASiCS using gene expression measurements from mouse Embryonic Stem Cells.
>back to "Talks and Speakers"
Recent Posts
- MLPM2012 has ended – thank you!
- Final ITN meeting and Krupp Symposium in Munich
- MLPM ITN fellow Melanie brings science to classrooms and inspires with simple but exciting experiments
- ESHG Symposium – a great success!
- Team working event: The 2nd ITN March retreat
Archive
2017
- January (1)
2016
2015
2014
2013
Categories
- Awards (2)
Authors
- Melanie (1)
- Felipe (1)
- MLPM-Admin (22)
- MLPM (2)
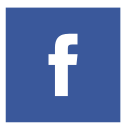
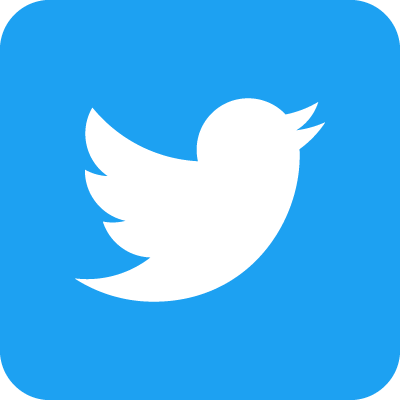