Probabilistic modelling of omic time course data
by Magnus Rattray
We are building models of the kinetic processes regulating the production of mRNA using time course data from high-throughput genomic technologies. We use non-parametric Gaussian process models to represent time-varying concentrations or activities and we integrate these non-parametric models with simple mechanistic difference [2] or differential equation models [1]. Parameter estimation and model-based prediction is carried out using Bayesian inference techniques. We use these models to help understand what are the rate-limiting steps in gene expression and how these processes are regulated.
References:
[1] Honkela, A, et al. "Genome-wide modelling of transcription kinetics reveals patterns of RNA processing delays." Proceedings of the National Academy of Sciences USA (in press, 2015: preprint at http://arxiv.org/abs/1503.01081)
[2] wa Maina, Ciira, et al. "Inference of RNA Polymerase II Transcription Dynamics from Chromatin Immunoprecipitation Time Course Data." PLoS Computational Biology 10.5 (2014).
>back to "Talks and Speakers"
Recent Posts
- MLPM2012 has ended – thank you!
- Final ITN meeting and Krupp Symposium in Munich
- MLPM ITN fellow Melanie brings science to classrooms and inspires with simple but exciting experiments
- ESHG Symposium – a great success!
- Team working event: The 2nd ITN March retreat
Archive
2017
- January (1)
2016
2015
2014
2013
Categories
- Awards (2)
Authors
- Melanie (1)
- Felipe (1)
- MLPM-Admin (22)
- MLPM (2)
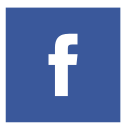
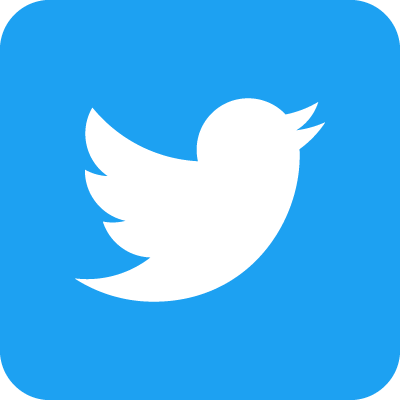