Modeling molecular heterogeneity between individuals and single cells
by Oliver Stegle
The analysis of large-scale expression datasets is often compromised by hidden structure between samples. In the context of genetic association studies, this structure can be linked to differences between individuals, which can reflect their genetic makeup (such as population structure) or be traced back to environmental and technical factors. In this talk, I will discuss statistical methods to reconstruct this structure from the observed data in order to account for it in genetic analyses. These approaches allow to accurately map the genetic determinants of high-dimensional molecular traits, including gene expression levels. By incorporating principles from causal reasoning, we show that critical pitfalls of falsely explaining away true biological signals can be effectively circumvented. In addition to applications in genetics, I will give an outlook how very similar approaches can be applied to model heterogeneity in single-cell transcriptome datasets.
>back to "Talks and Speakers"
Recent Posts
- MLPM2012 has ended – thank you!
- Final ITN meeting and Krupp Symposium in Munich
- MLPM ITN fellow Melanie brings science to classrooms and inspires with simple but exciting experiments
- ESHG Symposium – a great success!
- Team working event: The 2nd ITN March retreat
Archive
2017
- January (1)
2016
2015
2014
2013
Categories
- Awards (2)
Authors
- Melanie (1)
- Felipe (1)
- MLPM-Admin (22)
- MLPM (2)
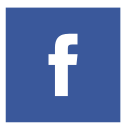
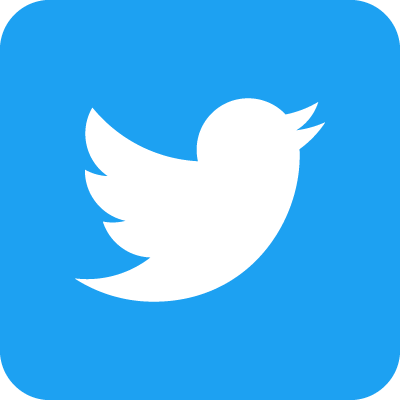