Heritability-based models for prediction of complex traits
by David Balding
Complex trait genetics has been revolutionised over the past 5 years by developments related to the concept of heritability. Heritability is the fraction of phenotypic variation that can be attributed to genetic mechanisms (mostly we focus on narrow-sense heritability, which considers only additive genetic effects). Since we cannot identify and measure the causal genetic mechanisms, a traditional approach has been to use pedigree relatedness as a proxy for the sharing of causal alleles between individuals. Pedigree relatedness even came to be seen as central to the concept of heritability, which perhaps explains why it was not until 2010 that it became widely appreciated that genome-wide genetic markers (SNPs) offered at least a "noisy" way to directly measure causal alleles, and hence a new approach to assessing heritability. This approach is "noisy" because SNPs generally only tag causal variants imperfectly, depending on SNP density and linkage disequilibrium, and many SNPs may tag little or no causal variation. So genome-wide SNP-based heritability estimates are difficult to interpret, but they can provide a lower bound which was enough to show that SNPs usually tag much more causal variation than can be attributed to genome-wide significant SNPs. Another big step forward has been that heritability can be attributed to different genes, genomic regions or functional classes, and for many phenotypes it is found to be widely dispersed across the genome, with relatively little concentration in coding regions. Further, heritability has become a unit of common currency for gene-based tests and meta-analysis. I will review the ideas and the underlying mathematical models, and present some recent results.
>back to "Talks and Speakers"
Recent Posts
- MLPM2012 has ended – thank you!
- Final ITN meeting and Krupp Symposium in Munich
- MLPM ITN fellow Melanie brings science to classrooms and inspires with simple but exciting experiments
- ESHG Symposium – a great success!
- Team working event: The 2nd ITN March retreat
Archive
2017
- January (1)
2016
2015
2014
2013
Categories
- Awards (2)
Authors
- Melanie (1)
- Felipe (1)
- MLPM-Admin (22)
- MLPM (2)
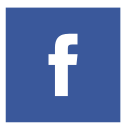
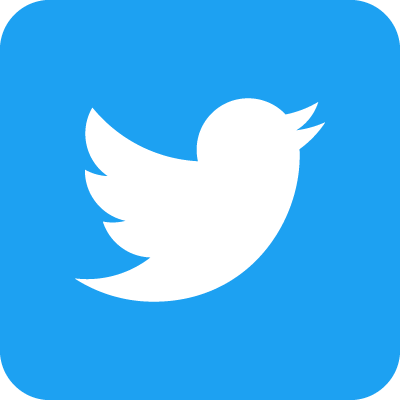