Computational Decision Theory (Model Misspecification)
by Chris Holmes
Bayesian analysis is rooted in decision theory following the axiomatic construction of Savage (1954). Decision theory provides a prescriptive approach to taking actions in the face of uncertainty and partial knowledge. As such it is ideally suited to biomedical machine learning applications, particularly those involving decision making from large-scale noisy data. In this talk we highlight the fundamentals of decision theoretic analysis through the minimization of expected loss in a number of biomedical applications. We pay particular attention to recent work in the sensitivity and robustness of inference and decision analysis to issues of model misspecification.
>back to "Talks and Speakers"
Recent Posts
- MLPM2012 has ended – thank you!
- Final ITN meeting and Krupp Symposium in Munich
- MLPM ITN fellow Melanie brings science to classrooms and inspires with simple but exciting experiments
- ESHG Symposium – a great success!
- Team working event: The 2nd ITN March retreat
Archive
2017
- January (1)
2016
2015
2014
2013
Categories
- Awards (2)
Authors
- Melanie (1)
- Felipe (1)
- MLPM-Admin (22)
- MLPM (2)
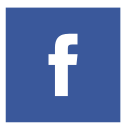
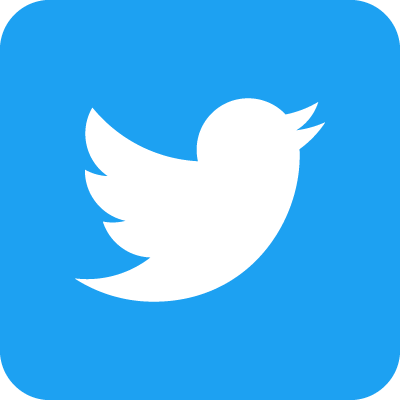