Project: Stochastic modelling and graphical models for the analysis and prediction of phenotype interactions
![]() |
![]() |
![]() |
Researcher: Melanie Fernandez Pradier |
Supervisor: Fernando Perez Cruz |
University Carlos III Madrid Madrid, Spain |
Melanie F. Pradier was born in Madrid, Spain, in 1986. She studied Telecommunication Engineering at the Technical University of Madrid (UPM), and obtained her MSc in Information Technology at the University of Stuttgart (Germany) in 2011. Her master thesis dealt with emotion recognition in speech signals and perception of music. Before starting her PhD, she spent almost 2 years working in diverse Machine Learning research projects in the industry, at Sony Corporation in Germany and Japan.
Project description
This project aims at designing statistical/machine learning models that help doctors better understand diseases and their complex interactions. Our current focus lies on the analysis of psychiatric disorders and their underlying interactions. Indeed, psychiatric disorders are known to have a high comorbidity, i.e., different disorders tend to co-occur at a higher frequency than chance. The problems that we are considering are the following:
- be able to detect the different psychiatric patterns.
- model the existing correlation across observations given a certain disorder.
- infer the respective relationships between different disorders.
All these problems are being addressed following a Bayesian nonparametric approach. Concretely, we consider both latent mixture models with Dirichlet Processes (DP), as well as latent feature models, using Indian Buffet Processes (IBP) as a prior.
About Bayesian Nonparametric Models:
Bayesian nonparametrics (BNP) has been an active field of research in the last decade. Such models have been used to address a wide variety of problems, such as classification, regression, clustering or collaborative filtering.
The Bayesian approach allows us to include all the available information a priori, which makes our model more robust, interpretable, and consistent with reality. Additionally, the Nonparametric property makes such methodology very flexible, for the solution complexity depends on the available amount of data.
If we have little data, the number of parameters will be small and simple solutions will be found, avoiding overfitting. But if many input samples are available, the nature of the problem will be more complex, and the number of parameters will automatically grow as necessary, avoiding model selection. This feature allow us to explain novel aspects of the data that were so far unknown.
Information about the used Database:
For this purpose, we have a large amount of information in the National Epidemiologic Survey on Alcohol and Related Conditions (NESARC) database. This database collects data on the background of participants, alcohol and drug usage, behavioral and personality traits. We are currently using Wave 1 of this database and we are trying to gain access to Wave 3 in which there is genetic information for the subjects.
Motivation for participating in the network
In the past decades, the emergence of new technologies has lead us to a true data explosion in all areas, including the field of medicine. Although we are able to collect a huge amount of data from patients, there is still a long path until we can process all this valuable information to provide a truly personalized medical treatment. My objective is to contribute to the understanding of diseases and their complex interactions by designing probabilistic models and statistical inference methods.
****************************
Duration of fellowship: from March 2013 to February 2016.
Contact: melanie@tsc.uc3m.es
Website: www.melaniefpradier.work
MLPM Publications
ORAL PRESENTATIONS (plus posters)
- Pradier MF, Moreno PG, Ruiz FJR, Valera I, Mollina-Bulla H, Perez-Cruz F.Map/Reduce Uncollapsed Gibbs Sampling for Bayesian Non Parametric Models. Spotlight Talk at Workshop in Software Engineering for Machine Learning at Neural Information Processing Systems Conference 2014 (NIPS2014).
- Pradier MF, Vogt JE, Stark S, Karaletsos T, Perez-Cruz F and Rätsch G. Probabilistic Analysis of Genetic Associations with Clinical Features in Cancer. Presented as Spotlight talk at the 9th Annual Machine Learning Symposium at New York Academy of Sciences, New York 2015.
POSTERS
- Pradier MF, Olmos PM, Perez-Cruz F. Lossy Source Compression of Multiple Gaussian Sources. European School of Information Theory 2013 (ESIT2013).
- Vogt JE, Pradier MF, Hyland S, Stark S, Lehmann K, Karaletsos T and Rätsch G. Clinical Notes, Sentence Clusters & Somatic Mutations. Poster at Workshop in Machine Learning for Clinical Data Analysis, Healthcare and Genomics at Neural Information Processing Systems Conference 2014 (NIPS2014).
- S. Stark, J. E. Vogt, M. F. Pradier, and G. Rätsch. Large-Scale Clustering of Sentences and Patients based on Electronic Health Records. Presented at the 9th Annual Machine Learning Symposium at New York Academy of Sciences, New York 2015.
AWARD
2015 Spotlight Talk Award for
Pradier MF, Vogt JE, Stark S, Karaletsos T, Perez-Cruz F and Rätsch G. Probabilistic Analysis of Genetic Associations with Clinical Features in Cancer. Presented at the 9th Annual Machine Learning Symposium at New York Academy of Sciences, New York 2015.
The Spotlight Talk Award recognized a series of the best oral research presentations delivered by early career investigators during the Symposium.
Other publications
- M. F. Pradier, P. M. Olmos, and F. Perez-Cruz, Entropy-Constrained Scalar Quantization with a Lossy-Compressed Bit. Submitted to IEEE Transactions on Information Theory.
Recent Posts
- MLPM2012 has ended – thank you!
- Final ITN meeting and Krupp Symposium in Munich
- MLPM ITN fellow Melanie brings science to classrooms and inspires with simple but exciting experiments
- ESHG Symposium – a great success!
- Team working event: The 2nd ITN March retreat
Archive
2017
- January (1)
2016
2015
2014
2013
Categories
- Awards (2)
Authors
- Melanie (1)
- Felipe (1)
- MLPM-Admin (22)
- MLPM (2)
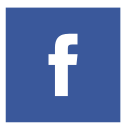
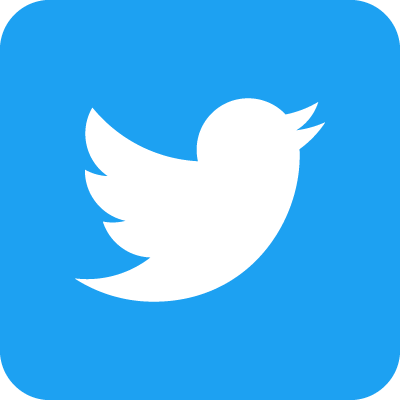