Project: Robust molecular signatures for personalized medicine
![]() |
![]() |
![]() |
Researcher: Yunlong Jiao
|
Supervisor: Jean-Philippe Vert |
Mines ParisTech Paris, France |
Yunlong Jiao was born in Hebi, China, in 1989. He studied Mathematics for Life Sciences at the Université Paris-Sud XI (France), and obtained his MSc in 2013 working on statistical modelling of breast cancer prognosis.
Project description
Researchers have been using machine learning techniques to detect molecular signatures in genomic data analysis in the past decade. However, the signatures developed from (pure) algorithmic points usually give no clear biological meaning, thus making a posteriori interpretation of the underlying results impractical. In order to overcome this limitation, there is a pressing need to use available biological networks from (public) databases to enforce biological relevance in the process of data analysis. In our study, given that a biological network is a priori provided, we try to capture the topological structure of the network to enhance the analysis of breast cancer prognosis.
Motivation for participating in the network
It was my passion for mathematics and biology since senior high school that motivated me to study bioinformatics. ITN offers great training for my career as a researcher, especially at the interface of machine learning and biology, and valuable opportunity to accumulate research experience via internships in both academic and industrial secondments.
************************************
Duration of fellowship: September 2013 to September 2016.
Contact: yunlong.jiao@mines-paristech.fr
Publications
ARTICLE
Jiao Y and Vert JP. The Kendall and Mallows Kernels for Permutations. Proceedings of the 32nd International Conference on Machine Learning (ICML), JMLR: W&CP 37, 1935-1944, 2015.
Eduati, F., Mangravite, L. M., Wang, T., Tang, H., Bare, J. C., Huang, R., ... & NIEHS-NCATS-UNC DREAM Toxicogenetics Collaboration. (2015). Prediction of human population responses to toxic compounds by a collaborative competition. Nature biotechnology.
ORAL PRESENTATION
Bernard E, Scornet E, Jiao Y, Stoven V, Walter T, Vert J-P. Kernel bilinear regression for toxicogenetics. NIPS Workshop on Machine Learning in Computational Biology (MLCB), Lake Tahoe, Nevada, December 2013.
Recent Posts
- MLPM2012 has ended – thank you!
- Final ITN meeting and Krupp Symposium in Munich
- MLPM ITN fellow Melanie brings science to classrooms and inspires with simple but exciting experiments
- ESHG Symposium – a great success!
- Team working event: The 2nd ITN March retreat
Archive
2017
- January (1)
2016
2015
2014
2013
Categories
- Awards (2)
Authors
- Melanie (1)
- Felipe (1)
- MLPM-Admin (22)
- MLPM (2)
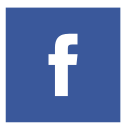
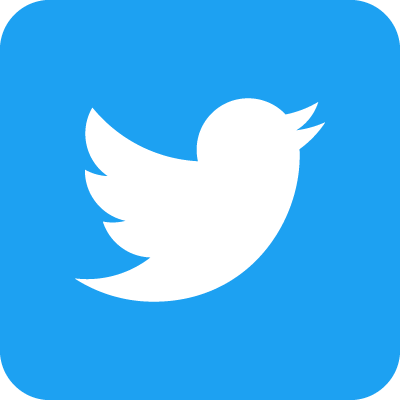