Project: Patient subtype discovery from heterogeneous datasets via unsupervised machine learning
![]() |
![]() |
![]() |
Researcher: Menno Witteveen |
Supervisor: Karsten Borgwardt |
Department of Biosystems Science and Engineering, ETH Zürich, Switzerland |
Menno Witteveen was born in The Hague, the Netherlands, in 1988. He studied Biotechnology at Wageningen University obtaining his BSc and continued his studies at the Technical University of Delft obtaining his MSc in computer science in 2014 working on “Identification and Elucidation of Expression Quantitative Trait Loci (eQTL) and their regulating mechanisms using Decodive Deep Learning.”
Project description
Although many genetic factors of disease have been discovered since the advent of next generation sequencing, much of the so-called missing heritability remains elusive. It is for instance now known that many diseases that were previously considered to be one specific disease are in fact a myriad of specific disease subtypes, each requiring a tailored treatment regimen. One of the crucial goals of personalized medicine is therefore to discover these disease subtypes, hopefully leading to better treatments.
Therefore this project is focused on the improved classification of patients into relevant disease subphenotypes. This will be done by integrating large-scale ‘omics’ datasources with other clinical parameters. The aim is to process these datatypes in order to identify the most suitable features to predict subphenotype group membership for individual patients.
Motivation for participation in the network
I believe the rapid development in sequencing and auxiliary technologies is going to revolutionize medicine and radically change our lives. I believe an interdisciplinary training environment at the interface of Machine Learning & Medicine will put me in the perfect position to contribute to this revolution. On this interface the challenge of improving classification of patients into clinical sub-phenotypes is really my main interest and I know that addressing structural aspects of this data will be vital to achieve this goal. This is the problem I am devoting myself to!
I have a predisposition to search for solutions that defy standard conventions, taking an interdisciplinary approach to heart. I enjoy new and different visions and perspectives from others and I am thrilled by the idea of studying abroad, meeting new people from the MLPM Network and beyond to inspire and to be inspired by. Because of my experience I now know that finding new statistical & computational approaches to deal with large and complex data problems is something I love to do and I have a strong conviction that, with evermore data around, the right algorithm, elegantly placed in its biological context, will make the difference.
****************************
Duration of fellowship: from November 2014 to December 2016
Contact: menno.witteveen@bsse.ethz.ch
MLPM2012 Publications:
ARTICLE
Roqueiro D*, Witteveen M*, Anttila V, Terwindt G, van den Maagdenberg A, Borgwardt K. In-silico phenotyping via co-training for improved phenotype prediction from genotype. Bioinformatics 2015, accepted.
(* equal first authors)
CODE
http://www.bsse.ethz.ch/mlcb/research/bioinformatics-and-computational-biology/co-training.html
ORAL PRESENTATION
Witteveen M. In silico phenotyping via co-.‐training for improved phenotype prediction from genotype. Retreat of the MTB graduate school, November 1st–3rd 2015, Kartause Ittingen, Switzerland
POSTER PRESENTATION
Roqueiro D*, Witteveen M*, Anttila V, Terwindt G, van den Maagdenberg A, Borgwardt K. In-silico phenotyping via co-training for improved phenotype prediction from genotype. Clinical Genomic Analysis Workshop, June 7, 2015, Haifa, Israel (* equal first authors)
Other publications:
- Plesa C, Ruitenberg JW, Witteveen MJ, Dekker C. Detection of Single Proteins Bound along DNA with Solid-State Nanopores. Biophysical Journal 2014; 106(2): 395a.
- Plesa C, Ruitenberg JW, Witteveen MJ, Dekker C. Detection of individual proteins bound along DNA using solid state nanopores. Nano Letters 2015.
- Plesa C, Verschueren D, Ruitenberg JW, Witteveen MJ et al. Observation of DNA Knots Using Solid-State Nanopores. Biophysical Journal 2015; 108(2): 166a.
Recent Posts
- MLPM2012 has ended – thank you!
- Final ITN meeting and Krupp Symposium in Munich
- MLPM ITN fellow Melanie brings science to classrooms and inspires with simple but exciting experiments
- ESHG Symposium – a great success!
- Team working event: The 2nd ITN March retreat
Archive
2017
- January (1)
2016
2015
2014
2013
Categories
- Awards (2)
Authors
- Melanie (1)
- Felipe (1)
- MLPM-Admin (22)
- MLPM (2)
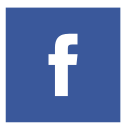
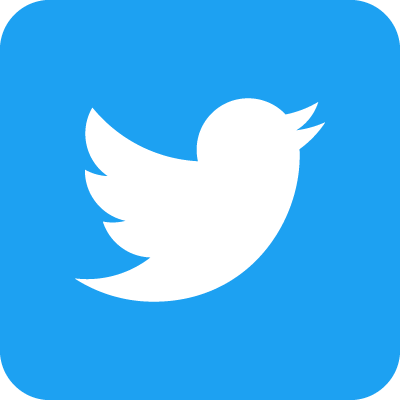